Your UAS flight management system affects accuracy in photogrammetry, so get to know what you need.
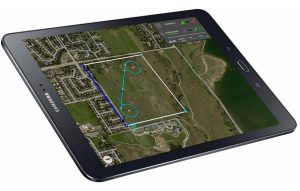
Black Swift’s Swift Core Flight Management System is comprised of the SwiftPilot autopilot, the tablet UI (shown here), and the ground station.
Unmanned aircraft systems (UAS) are arguably the most disruptive technology introduced to the geospatial profession since GPS. Advances in UAS capabilities and in photogrammetry software have significantly reduced the barrier to generating digital surface models (DSM) and orthomosaics from geo-referenced aerial imagery.
Advancements in cameras, sensors, and airframes have made it possible to achieve less than 5cm in vertical error (when using a fixed-wing UAS) with precision RTK GPS position measurements.
Yet, in spite of these advancements, it is the flight management system (FMS) or autopilot system that operators use to plan and execute their mission that is critical to the success of the data collection. These autopilot systems represent a fundamental element that can influence, positively or negatively, the accuracy of DSM achieved with a drone using photogrammetry.
Flight precision is essential to obtaining the accuracy required for aerial land surveying, elevation mapping, or photogrammetry. With FMS playing such a critical role in the accuracy and precision of your data capture, what should you look for when evaluating autopilot systems available today?
A variety of elements affects the effectiveness of your autopilot system and, in turn, the reliability and accuracy of your final data set. These include density and consistency of image overlap, calibration of mid-exposure time of photos taken, accuracy of the image sets taken, extent of sensor/filter integration, overall platform connectivity, and the ease of flight planning and execution.
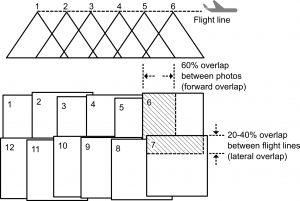
Figure 1: Overlapping photo pattern required for photogrammetry. (Image modified from a drawing on Resource Canada’s website.)
Uniform Image Overlap
A typical photogrammetry mission is based on the UAS flying along pre-planned flight paths using GPS waypoints. Along the path, the vehicle takes multiple overlapping photos with typical photogrammetry requiring at least 60% forward overlap (Figure 1).
Overlap is defined as the amount by which one photograph includes the area covered by another photograph and is expressed as a percentage. Side overlap, or sidelap, in traditional aerial photogrammetry is suggested to be around 20% to 30%; however, for accurate elevation models the sidelap should match the forward overlap. Further, high-accuracy DSM generation requires overlap and sidelap closer to 80% to 90%.
Uniform image overlap, with a density of at least 10+ images overlapping, is critical for any system to capture precise and usable survey-quality data. The ability for an aerial platform to maintain a regular overlap pattern is essential for accurate data processing, particularly to resolve the shape of the terrain and contained structures.
Irregular patterns can result in gaps in the 3D data sets, compromising the accuracy of the data captured (Figure 2). If the gap is near a control point or is in a location that is to be used by the survey application, it’ll need to be reflown, which can be costly and frustrating for the operator.
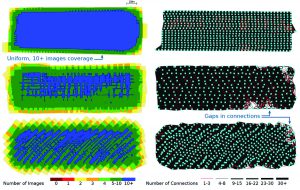
Figure 2: Examples of coverage maps and the connected mesh.
Photo Timing
The ability to measure mid-exposure time to provide an accurate time-based signal from the camera is essential. Equally important is the ability of the autopilot to accurately measure the arrival time of the pulse from the camera.
However, some autopilot systems use a method of polling to detect the state of digital input/output lines. In a polling method, the accuracy of the time is limited by the update rate of the polling loop.
More advanced systems use an interrupt-driven architecture and thus are able to detect the trigger down to microsecond resolution, resulting in superior results.
While timing is important, it is not error-free. If you base your image triggers strictly on timing and you fly tracks into and with the wind, one direction will have images spaced closer than the other direction.
Accuracy of Image Positioning
Inconsistencies between image sets (image position estimated by autopilot and actual image position) can affect not only absolute accuracy of your data but also the amount of processing time required for gleaning usable data from your image sets (Figure 3).
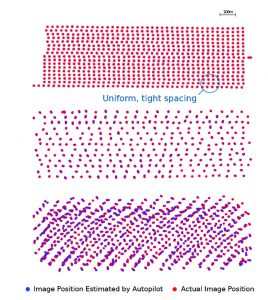
Examples of actual image position compared to calculated image position.
Accurate autopilot geo-tagging requires uniform image sampling and maintaining proper orientation of the aircraft and its payload during image capture. Third-party geotagging solutions can introduce errors in orientation estimates, even if they have an accurate RTK-based location solutions, as it’s difficult to estimate the orientation of the aircraft without a full-sensor suite (specifically, wind measurements for all platforms and airspeed for fixed-wing platforms).
More importantly, the re-projection errors are propagated through data analysis and will result in longer processing time as well as larger errors when producing geo-rectified orthomosaics and terrain information. Both can result in higher costs incurred per data set, either in processing time, which can be expensive, or by requiring a re-deployment to gather a second data set without the large errors.
Flight Planning
Experience shows that a careful design of the aircraft trajectory (waypoints, strips, speed, attitude, etc.) and a flexible real-time mission management capacity (sensor configuration, triggering events, flying directions, etc.) are instrumental in achieving robust and reliable data-collection missions.
One of the most common problems with many autopilot systems is that they do not generate proper flight plans for various types of aircraft. Especially because they don’t account for the dynamics constraints of the vehicle or the atmospheric conditions encountered (e.g., wind), the aircraft has a difficult time tracking the flight plan. This results in reduced sidelap and will negatively affect the accuracy of a final DSM.
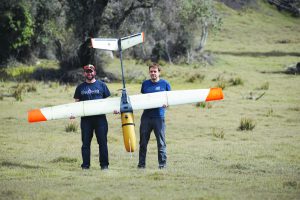
Black Swift’s S2 UAS during volcano monitoring missions earlier this year in Costa Rica. Holding the aircraft are Jack Elston (left) and Maciej Stachura.
Ease of Use
When time is of the essence, having a flight management system that is intuitive and easy to program becomes paramount. Tablet-based autopilots provide the mobility operators demand while also supporting an interface users are familiar with (e.g., gesture-based navigation).
This means that operators can easily calculate the area of interest for review and begin the process of collecting data for immediate analysis and decision-making. A robust system should allow you to monitor your mission and mapping directly from your tablet.
Repeatability
Systems that require frequent software updates are difficult to use for a variety of reasons. In remote field locations operations can be inhibited if the UI is updated but the autopilot isn’t and connectivity to the internet is not available.
Additionally, it’s difficult to guarantee consistency between data sets when each was flown with a different version of control or UI software. This is particularly a problem when attempting to perform change detection.
Integrated Solution
A GPS-enabled ground control station with wireless connectivity (900MHz radio, for example) as well as application-specific sensor integration is necessary to ensure the most accurate and seamless data capture available. This sensor-based approach to flight planning and management optimizes the quality of the observed data by autonomously modifying the flight path based on sensor inputs. The result is that users can collect data over geography that is topically diverse.
Holistic V. Individualized Approach
Given the potential market value of a UAS platform able to produce accurate DSMs on demand with no ground control, there has been a significant push by the UAS industry to find a solution. However, given that companies in the UAS space rarely have an understanding or control over all components of the system, most approaches fail to consider the system as a whole.
This is particularly true about the autopilot system which is quite complex. Even in cases where the firmware is open source, sufficient expertise does not exist to determine the sources of error from the setup, let alone the knowledge to correct them. This has led to masking sources of error by jumping to the conclusion that a single, more-accurate sensor or additional subsystem will solve the accurate-DSM-generation problem.
Commercially available autopilots typically are customized for their specific platforms and payloads. These solutions, in contrast to open-source alternatives, typically employ higher quality components and manufacturing methods, resulting in significantly higher MTBF (Mean Time Between Failure).
Some also incorporate key safety elements such as double and even triple redundancy, as well as achieving Software Considerations in Airborne Systems and Equipment Certifications, such as DO-178B/DO-178C/ED-12B/DO-254, which authorities such as FAA, EASA, and Transport Canada approve.
Support
Another key differentiator between open-source and commercially available autopilot solutions is the level of technical support readily available. Commercial solutions typically provide technical support whereas with open-source alternatives users often rely on online forums.
Considering just the UAS, the ability to generate high-quality aerial data is based on (in order of importance) the autopilot system, the camera and lens combination, flight planning, and finally the vehicle. The aircraft, autopilot, and camera interface all have significant impact on the data obtained and can be the difference between a successful data collect and a costly repeat flight, or worse, the inability to complete the job as bid.