Artificial intelligence is making huge waves in the geospatial industry, changing how location information and BIMs are created from big data. Meet three startups at the forefront.
(Image above: By applying computer vision to street imagery, Mapillary detects map data at scale.)
Startups in the AEC realm have skyrocketed over the last decade. The availability of innovative technologies—GPS, satellites, UAVs, lidar, and photogrammetry—has driven their success and, as a result, has made more tools and data available to geospatial professionals. So if the 2010s was the decade of startups, the 2020s will be driven by artificial intelligence (AI).

Ecopia’s AI system can rapidly generate accurate and high-definition vector maps like this one from any geospatial imagery.
Why AI?
Think of big data, and this makes sense. The unprecedented deluge of data churned out by new geospatial devices can be efficiently organized and processed only using AI algorithms. Companies are now exploiting AI to extract real-world objects, from point clouds to satellite imagery. With AI technology, houses can be easily distinguished from forests, windows from walls, pipes from conduits, all with exceptional speed and precision.
However, AI must first train its algorithms to search for significant data patterns, and to do so requires terabytes of accurate data.
Even this is no longer a problem. As location and imaging technologies have become more accurate, the storage capacity of chips has also exponentially expanded, and cloud storage solutions have become so ubiquitous that geospatial professionals can easily capture and store high volumes of precision data with ease.
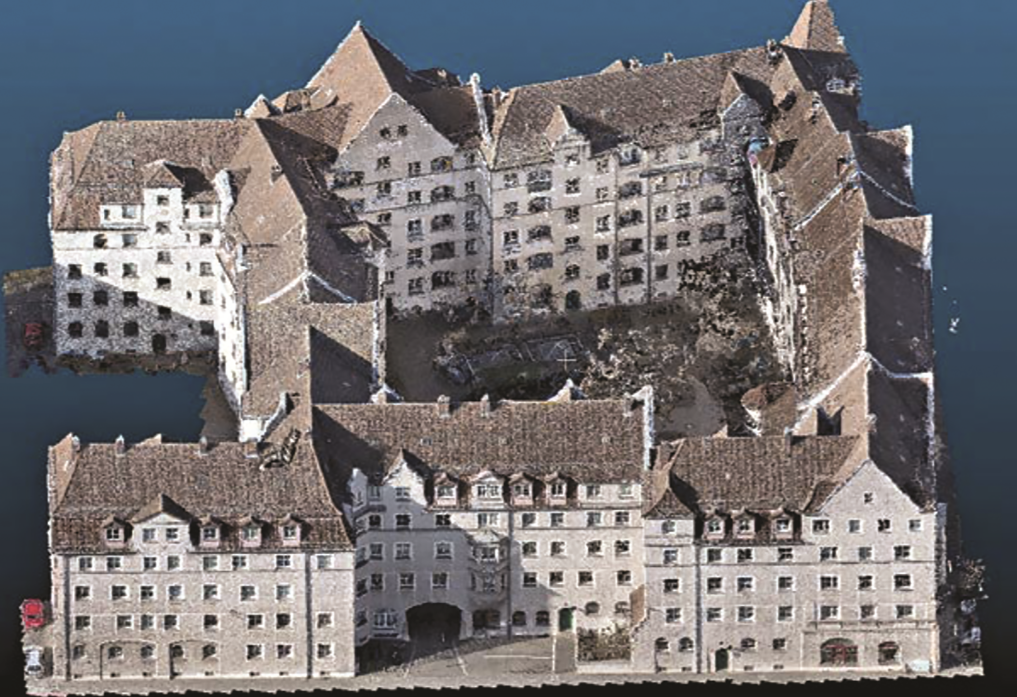
This point cloud of a staircase is from a large residential complex in Nuremberg created using VOXELGRID technology.
These days, the amount of information that you can collect with compact-sized gadgets is mind-blowing. Think of nano-satellites, palm-sized UAVs, and handheld 3D scanners, all feeding to AI’s hunger for data.
Thus, in the next decade’s era of big data, the new revolution in the geospatial industry will come from successfully using AI to unearth useful products from massive datasets. These geospatial startups are leading the way.
Improving Street-level Mapping with Computer Vision
What do you do when you realize that the map you need is not updated fast enough by big mapping companies? You create your own company to do just that.
Mapillary
In 2013, Jan Erik Solem and three others co-founded Mapillary, a startup from Sweden that uses computer vision technology to extract valuable data found in street-level imagery. Their novel mapping approach has received the nod of top-notch investors and companies; they now collaborate with global companies such as HERE, Volkswagen, and Amazon.
Computers are able to detect and position objects from images at a much faster pace and a more detailed level than any human could accomplish. Now, Mapillary has become the world’s largest publicly available image database for improving maps, all thanks to AI.
“AI technology has been the cornerstone of Mapillary from the very beginning,” says Solem, CEO. “With computer vision, a form of artificial intelligence, machines could see and, in the best case, understand what they’re seeing.”

VOXELGRID’s rec ording device can capture up to 10,000 square meters (2.5 acres) of floor area in a day.
Mapillary’s computer vision technology has been trained to detect objects like traffic signs, fire hydrants, utility poles, and many other objects in street-level imagery and then position this information on a global map.
Detailed Approaches
Playing on the words “map” and “capillary,” the company aims to reach the smallest ends of the world (similar to the smallest blood vessels in your body) and bring that scalability to mapping.
“We work mainly with three sectors: mapping, automotive, and players who need maps to be able to operate but don’t necessarily have their own mapping team,” says Solem.
For example, Mapillary helps companies to capture images as they go about their day-to-day operations and then use that imagery to cut the time they spend on things like finding parking and the right building entrance.
It also helps them with route optimization. In October 2019, Mapillary launched its own mapping dashcam with the aim to help logistics companies have access to near real-time map and location data through the Mapillary platform.
AI Easy?
Although AI technology may seem out of reach for the average map user, just about anyone can go on the Mapillary website and view the imagery and data at no cost. All imagery is available under the CC-BY-SA license, meaning they can be shared, used, and built upon, and the derived map data are freely available for editing in OpenStreetMap.
Mapillary believes that map users can benefit from its computer vision technology not just by being open, but also because of the speed and scalability that their technology brings.
“The need for map data is booming as map companies scramble to access up-to-date data in a growing number of markets. At the same time, the combination of increasing pressure on delivery firms and the rise of last-mile and micro-mobility solutions means that more players than ever before need access to map data,” says Solem. “Mapillary addresses this growing need of keeping maps updated by allowing everyone to map what is important to them just by capturing images.”
The problem with Easy
Allowing just about anyone to contribute images may have its drawbacks, including issues on data accuracy and privacy. So how does Mapillary deal with these issues?
“Accuracy is something we will always strive to improve, and as our dataset grows, our computer vision algorithms improve along with it,” says Solem. “We also use a technique known as ‘human in the loop’ or HOOP, where community members help us improve the accuracy of our detections by verifying whether the detections are correct or not.”
According to Solem, protecting privacy is a top priority for the company. “From the beginning, it was clear that we needed a way to anonymize sensitive information like faces and license plates in the images on our platform. Today privacy blurring is something we do with 99% accuracy, an industry best,” he says.
No Problem with Problems
With the expected growth of businesses that rely on location technology in the next decade, expect to see the use of AI technology in the mapping industry to go mainstream.
“These days it’s not just humans that need maps; it’s cars, delivery robots, last-mile solutions, and much more,” says Solem. “The market for maps for autonomous vehicles alone is set to grow from $2.2bn in 2020 to $24.5bn by 2050, so it’s safe to say that we’re just at the beginning of a big shift in how the world thinks about maps.”
For now, sustaining the success of the company will depend on the collaborative mapping platform that Mapillary has built.
“As more images are contributed by users, the more training data we can use to improve our AI algorithms, eventually resulting to the best mapping data available for everyone,” adds Solem. “As we say at Mapillary, collaboration wins.”
Creating Cost-effective 2D Plans & BIMs with Deep Learning
“It’s our magic sauce,” says Christian Wetzel, the man behind German start-up VOXELGRID, referring to how he believes his company can create cost-effective 2D plans and BIMs of existing buildings better than other companies.
VOXELGRID
This reduction in cost is important to customers, especially among government agencies and city planners. VOXELGRID’s economical approach has already been tested with the German government agency that manages the country’s properties (the Institute for Federal Real Estate), as well as with several municipalities in the country, to deliver building floorplans and floor-material information at lower costs.
“As an example, we did the whole façade of a large residential unit in Nuremberg, proving that with our methodology we can go from the quick estimation of the building’s area using deep learning up to its very detailed 3D reconstruction,” says Wetzel. “And we did it at a cheap price.”
Algorithms: not just for evil
Founded in 2016, VOXELGRID’s technology uses novel AI techniques, including automatic area recognition and deep learning, to accomplish point-cloud processing and hyperspectral analysis. The startup’s algorithms are so robust that it can automatically detect window areas from facades with less than 1% area deviation in 1 second, a very high level of precision by industry standards.
“Existing buildings without reliable planning documents account for around 95% of the entire global real estate portfolio,” says Wetzel. “The company’s focus is to supply combined hardware and software as a service (H&SaaS) in order to create layouts and material information for existing buildings which are way cheaper than traditional approaches.”
Creating a Record
VOXELGRID has also introduced to the market its own recording device, allowing professionals to capture up to 10,000 square meters (2.5 acres) of an existing building’s floor area in a day. With little post-processing effort, 2D plans or a 3D building model with information on its materials can be generated automatically.
“Compatible and accurate building data is a prerequisite for creating BIMs. Our goal is to provide building information, including room number, height, and its materials, inside and out,” says Wetzel.
At the heart of VOXELGRID’s process is AI technology. “Using hundreds of annotated building images as training datasets, we teach computers to do what comes naturally to humans: learn by example and experience. The outcome is a remarkable progress in image recognition of building geometries, especially in identifying different materials and components,” says Wetzel.
But isn’t this type technology used by others, as well?
“I think that there is currently no other company offering to create 2D plans and 3D models using this set of imaging technologies,” says Wetzel.
And he might be right. When most companies are featuring either the creation of point clouds or the virtual tour of a building, VOXELGRID differentiates itself by using its proprietary AI algorithms to quickly generate industry-compatible BIM data for existing buildings. Their combination of innovation and speed is more cost-effective in the long run, allowing them to offer more affordable products compared to their nearest competitors.
In addition to receiving awards, the company has been invested in by the CalCon Group, one of the largest IT companies in the German and Austrian real estate market. VOXELGRID now has a staff of 16 from the original three and continues to operate from Munich, the country’s startup hub in the south.
So with pilot projects and rounds of financing underway, what’s the next step for the company?
“The long-term goal is to develop realistic 3D building models that contain all the information about the geometries and structural elements,” shares Wetzel. “But we have to be really aware that proper AI only works with proper training, and this means really an awful lot of suitable information for training the machine.”
Digitizing the World with Machine-learning Processes
The Canadian company Ecopia.AI (Ecopia) created a buzz in the geospatial industry in 2018 when they released the first high-precision and complete building footprint dataset of the United States. Many people believed it could not be done at that scale, speed, and precision.
Ecopia
To achieve this, the company’s AI algorithms combed through Maxar’s DigitalGlobe satellite imagery database, extracting 169 million building footprints across the United States and updating it annually.
The company has taken on a bigger challenge this year: to create the first comprehensive digital map of all of Sub-Saharan Africa. This digital map will include an estimated 342 million buildings, double the size of what they created in the U.S.
“Ecopia leverages AI to mine geospatial big data. This data is used to create up-to-date, highly accurate digital representations of the Earth that are being used by decision-makers around the world,” says Emily Jackson, company vice president, communications. “We produce millions of kilometers worth of commercial-grade high-definition maps of the Earth every month.”
To support their Sub-Saharan Africa initiative, Ecopia received $6.7 million funding from the Sustainable Development Technology Canada (SDTC), a foundation created by the Canadian government.
Ecopia’s core technology was developed through the PhD research of Yuanming Shu at the University of Waterloo, a leader in computer engineering. During his research, Shu noted that millions of geospatial images are captured every day by satellites, airplanes, drones, and other vehicles. This presented him with an opportunity to do something meaningful with the available pixels and data.
In 2015, Shu co-founded and launched Ecopia with Jon Lipinski and Shuo Tan, now the company’s president and CTO, respectively. A grant from the University of Waterloo and Federal Government of Canada allowed the founding team to begin exploring business opportunities. Within a year, they had their initial prototype and first customer.
Asked whether the company’s initial location was also a catalyst for Ecopia’s growth, Jackson’s response was positive. “The Accelerator Centre in Waterloo allowed us to grow as a business with access to other startups to talk about growth and best practices. We also had strong mentorship from industry experts, and as a growing startup, that expertise was invaluable.”
Growing pains? Growing excitement!
Like many other startups, Ecopia has faced growth challenges, including access to talent, sustainable revenue, and customers. “These are the three biggest challenges for any new startup company, but we believed in our team, our business model, and our product,” shares Jackson.
Telecommunication firms, governments, NGOs, insurance companies, and logistics use their maps to determine where building footprints, roadways, greenspace and other features are located.
“There is an inadequate amount of accurate, up-to-date information of the Earth,” says Jackson. “Current sources do not provide adequate solutions for enterprise analysis and decision making.”
The company’s artificial intelligence technique is currently an industry leader, specializing in extracting information from big geospatial data.
“We are leading the charge in how AI can be used to create high-definition vector maps at scale,” says Jackson.
Their advancements in machine learning can convert high-resolution Earth imagery into vector maps with a production capacity of more than 40 million building footprints per month, an unmatched capability that maintains 95% accuracy.
In addition to their products, Emily tells us what else makes them proud to be startup. “We have doubled in size and revenue year over year since incorporating as a business, all while being primary bootstrapped and not taking on any outside funding,” she says. “We have big plans for international expansion and product-line growth.”
Unscrambling the Buzzwords
John McCarthy, computer scientist at Stanford University, first coined the term “artificial intelligence” in 1955, defining it as, “the science and engineering of making intelligent machines, especially intelligent computer programs.”
Machines are intelligent when they can replicate human cognitive skills such as forming categories, recognizing patterns, and solving problems.
For machines to achieve artificial intelligence, computer programmers must create algorithms that can scan and organize input data to train and teach the machines to perform tasks. This method is called machine learning, the most basic of all AI techniques.
Several other AI methods have since been developed, including neural network, a process that mimics how the human brain learns by using interconnected nodes to process data. Its more sophisticated version is called deep learning, which uses multiple layers of neural networks so that computers can analyze bigger datasets. With the advent of big geospatial data, there are far more inputs for AI machines to train on and improve their accuracy.
And due to AI’s ability to handle big datasets such as imagery, computer vision is currently one of the hottest geospatial applications. With computer vision technology, machines can be taught to “see” real-world objects from images, thus allowing companies like Mapillary to identify roads from street photos, Ecopia to extract built-up areas from satellite imagery, and VOXELGRID to recognize construction materials from a point cloud.