A string of high-profile bridge failures in recent years has underscored the importance of innovating the way we inspect and assess the condition of our vital civil infrastructure. Can the use of AI help prevent bridge collapses in the future? This award-winning engineer thinks so.
The period spanning 1992 to 2014 could just be the worst periods for America’s bridges. According to the database of the National Bridge Inventory (NBI), a total of 428 bridges collapsed in the U.S. during those two decades, and the majority of them were caused by structural defects.
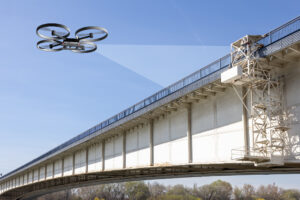
A quadcopter performs a bridge inspection by taking high-resolution digital images and scans.
For a country that prides itself on its long tradition of building monumental bridges, it is remarkable that more than 40,000 bridges in the U.S. are considered structurally deficient, including the famous Brooklyn Bridge of New York City. In addition, at least one in three bridges in the U.S. needs replacing or repairing, according to data from the Federal Highway Administration (FHWA), the agency that oversees the construction and maintenance of the nation’s highways and byways.
Protecting the safety of the public requires mandatory bridge inspections on a regular basis, normally at least once a year or every two years. This interval depends on various factors, such as the bridge’s actual condition, the volume of traffic that crosses it, as well as its structural system, location, and age. Important data are collected during these periodic inspections to guide preventive and conservative maintenance work and help keep bridges safe and reliable throughout their service life.
Unfortunately, the techniques to assess the structural soundness of bridges are still mostly traditional, involving trained inspectors performing visual checkups. As such, these manual and subjective inspections are often laborious, time-consuming, expensive, and, as in the case of bridges that span wide bodies of water or deep valleys, dangerous.
Dr. Ali Khaloo wants to change that. After a decade of work, including a job as a civil engineer inspecting and rehabilitating major infrastructure systems in some of the most active seismic zones in the world, and later obtaining a Ph.D. in structural engineering and a postdoc in artificial intelligence (AI) at Cornell University, Khaloo in 2019 co-founded Aren, a tech company based in New York City.
Aren provides AI powered solutions that can be used to safely and automatically detect a variety of structural deficiencies, such as pavement cracks and corrosion on bridges and other infrastructure assets. Khaloo’s patented AI technology has already won awards, including the coveted COINS Grand Challenge, a global competition that recognizes innovative ideas that aim to solve challenges facing the construction sector.
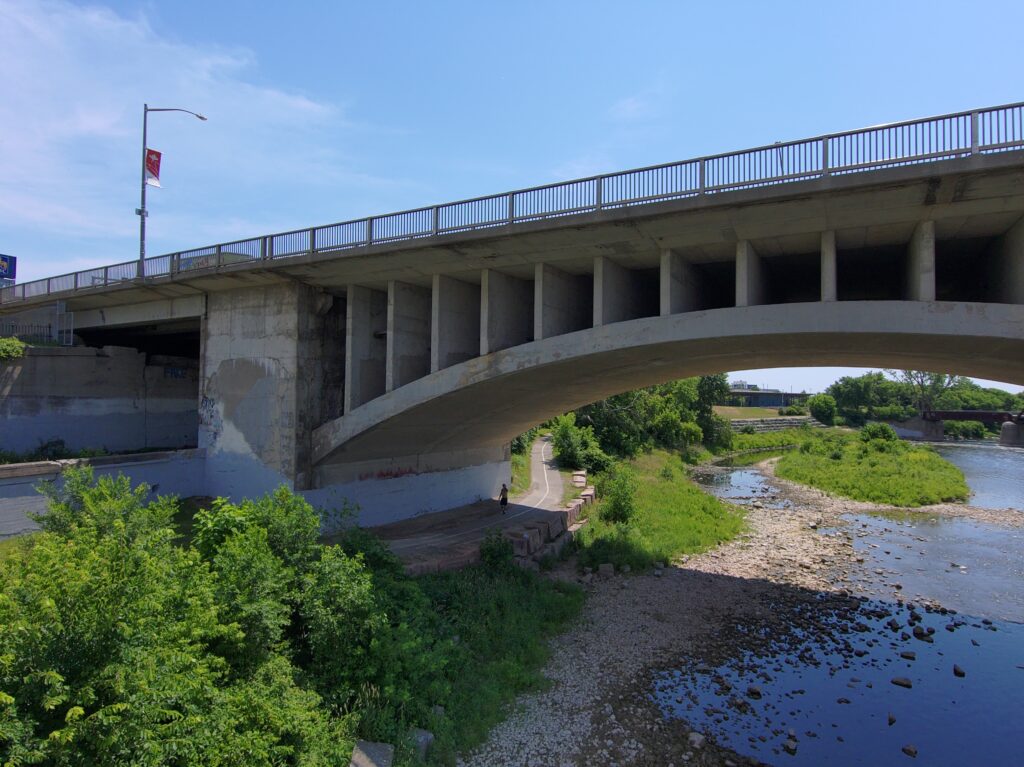
The Lorne Bridge in Ontario, Canada, where Aren used drones to capture the bridge’s imagery, which was then used to build its 3D digital twin.
In this interview, which has been edited for brevity and clarity, Khaloo shares with us his international experience in asset inspections, including how AI and image-capturing drones are now making bridge assessments more reliable. He also tells us why jobs of human bridge inspectors will not disappear any time soon.
How important is utilities and assets inspection for governments and private owners?
Inspection is the first line of battle when it comes to civil infrastructure asset management. This is a mandatory step to understand the condition of the assets. Depending on the type and condition of the asset, inspections are done at a certain frequency in order to track the possible changes in the assets and avoid their significant degradation.
What is your impression of civil assets management in the U.S.?
Current methods of infrastructure inspection, assessment, and management are precarious and outdated. Unfortunately, this is a global phenomenon. Currently, engineers walk, climb, and use snooper trucks to assess miles of infrastructure and use their naked eyes to identify damage and take notes of their findings on a piece of paper. Then they have to go through decades of old assessment reports to compare their findings and understand if the bridge is deteriorating or not.
This poor and insufficient information feeds maintenance recommendations and as a result, trillions of dollars in infrastructure budgets are poorly spent and people are getting killed. This problem is hitting the pockets of asset owners, consulting engineering firms, and insurance companies.
How then can utilities and assets inspection be improved in the U.S.?
While the current inspection process has a long track record, it isn’t perfect. It relies heavily on human judgment, so it is prone to human errors and variability. Over the years, several notable studies have shown that bridge ratings can vary widely from one inspector to another.
Inspector variances don’t necessarily lead to unsafe operating conditions, but it does make it challenging to use inspection data quantitatively as a part of an asset management program. It is difficult to quantify the aging process itself, given current standards. The inspection team inevitably changes over the life of a structure, adding a new source of variability and inconsistency to the inspection process.
What we need now are new data analytics tools that support engineers in this process. We need new technologies that fuse data together, integrate measurements, and provide a reliable and quantitative source of information.
And we need to be able to consider this data as a time series that changes as the asset ages. This is a considerable data science challenge, but the recent revolution in artificial intelligence has opened the door to new opportunities. That is exactly what we do at Aren by merging AI with civil engineering technology to overcome the current challenges with an innovative approach.
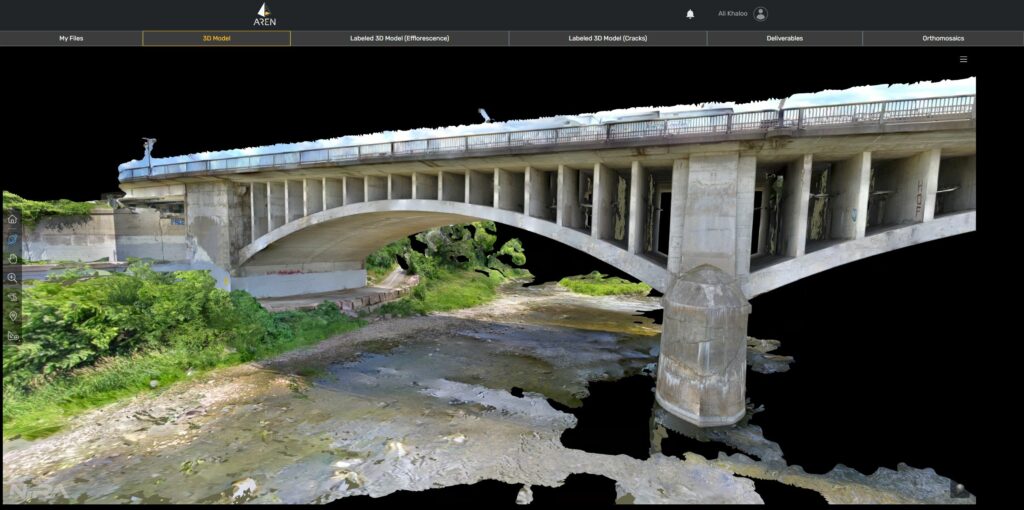
The digital twin of the Lorne Bridge as viewed using the Aren platform.
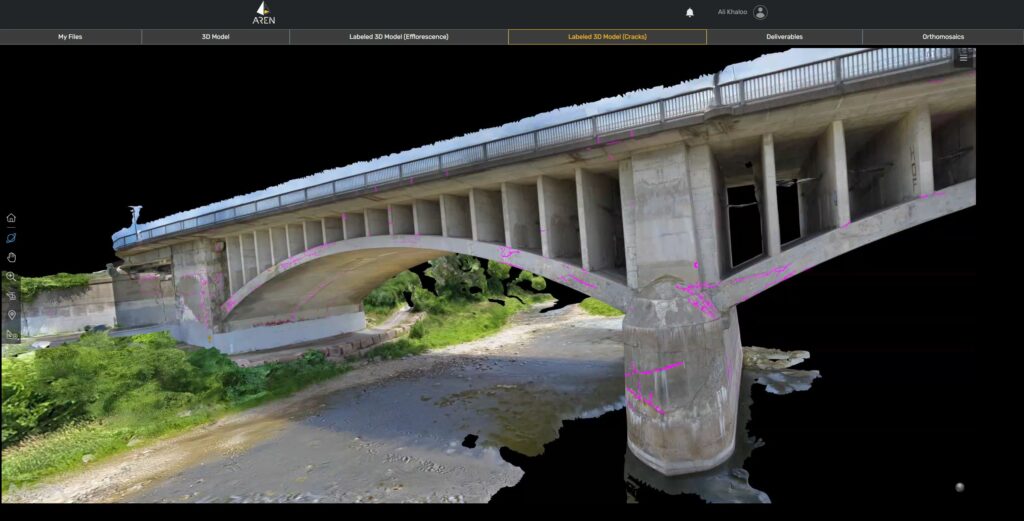
Cracks (in magenta) detected on the surface of the Lorne Bridge by the Aren platform.
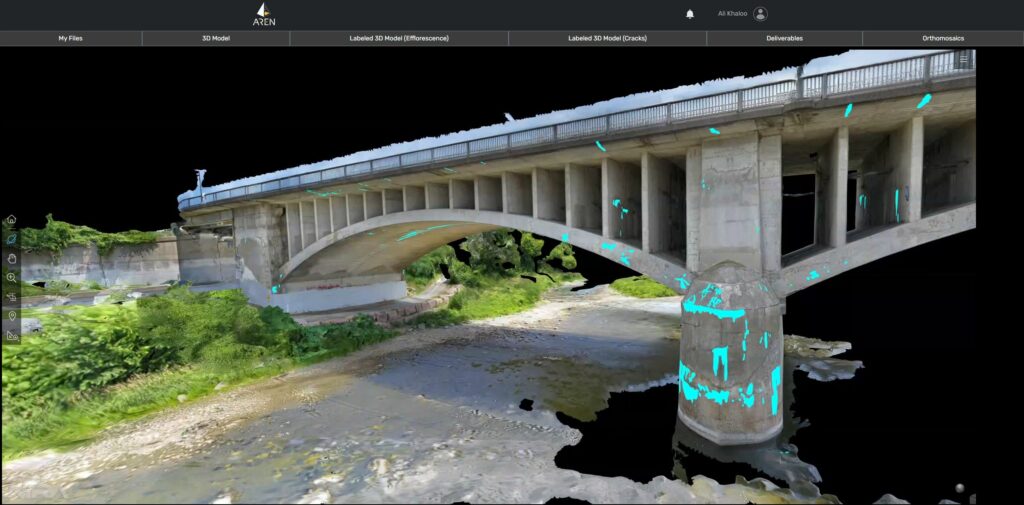
Efflorescence, or deposits of salts (in cyan), detected on the surface of the Lorne Bridge by the Aren platform.
In a nutshell, please tell us about Aren and how it started.
Aren came out of my years of experience as an engineer and later researcher. Even as a researcher, I was always trying to look for new and innovative solutions to solve some of the pain points I faced as an engineer.
For my Ph.D., I was looking for a scalable solution that can be applied to as many structures as possible. To do so, I got inspired by two things. First, the advancements in remote sensing technologies such as camera-equipped drones and even the quality of the cameras on our smartphones. Secondly was what I saw in the medical imaging industry in which they were pushing to use AI as a diagnostic tool to analyze MRIs and CT-Scans to find abnormalities. So, I started to bring these technologies into the realm of civil engineering and infrastructure assessment and management.
As I started to present my work at major conferences, I saw a huge interest from different stakeholders in the industry regarding applying this technology to their assets. We then started to get grants for proof-of-concept projects across the country. That was the time that I knew that my work could become the next big thing and the only way to push the industry forward is by bringing it to the market in an entrepreneurial fashion.
What was the most difficult challenge that you faced during those first days?
Bringing new and deep technologies to any market is difficult, let alone to the infrastructure and construction industry, which is one the least digitized industries in the world.
There was, and still is, a need to educate the market. The fact that we have a civil and structural engineering background helps a lot when we are talking to our customers since in their eyes, we know the status quo and its pain points pretty well. We literally try to change the industry from the inside out.
So how does Aren make use of AI technology to detect cracks or structural issues in infrastructures?
For more than 10 years we have built a technology that uniquely combines computer vision, machine learning, and civil engineering. Our platform automatically aggregates raw data from different sources, such as images, video, laser scanners, and other sensors. As outputs, it provides a 3D high-resolution visualization of the asset and its health through time, as well as predictive maintenance plans, capital allocation plans, and service life estimates.
In a nutshell, we build a digital health record of the assets. Upon receiving the data, we use our AI to detect a variety of damages in the assets. And not only do we detect them, but we also quantify them and provide a severity analysis. Also, as the data gets collected over different timestamps, we are able to assess the changes in time automatically.
How do you train your AI model to detect structural damage?
Training data of civil infrastructure assets and their damages are not available as a public dataset. For instance, in the autonomous vehicle industry you have many publicly available datasets to train models. Therefore, we had to curate a large proprietary training dataset over the years, which we have used to train our AI. In addition, we have an active learning pipeline that helps us to improve the accuracy of our models over time.
Tell us the places where you have successfully used Aren.
Thankfully, we are servicing clients in North America, several countries in Europe, and all the way to Japan. So far, we have worked on different asset classes such as bridges, buildings, dams, levees, tunnels, cooling towers, industrial chimneys, and airport runways.
For instance, we helped with the condition assessment of the Lorne Bridge in Ontario, Canada. There a separate team collected thousands of imagery data using Skydio drone in an autonomous fashion. The drone enabled us to get very close to the bridge and cover the entirety of it.
Using Aren’s technology, we first built a highly accurate and photorealistic 3D digital twin of the bridge to go from images to the full context of the asset. Later by using our AI we detected different types of damage that can happen on a concrete bridge, such as cracks, efflorescence, and spalling, some even submillimeter in size. All this damage was mapped into the 3D model to provide semantically labeled 3D models. Of course, all the damage was quantified, and we provided the severity analysis of each and every one of them.
How easy can drones be integrated within the workflows of Aren?
Very easily. And the good thing is that we are agnostic to the type of drone, whether fixed wing or quadcopters, as well as their cameras. We can analyze images in the range of hundreds of megapixels and more. Drones can provide a high-resolution visual characterization of an asset analogous to what is provided by the inspection process.
The improvements in camera sensors that can be carried by drones along with the advanced obstacle avoidance systems implemented in them make drones a great tool to augment the inspection process and gather gigabytes (and in some cases terabytes) of visual data, including RGB images and infrared. Of course, the natural next step is to analyze all this data automatically and that is where Aren comes into play.
What then is the typical Aren workflow when drones are used to inspect infrastructure?
We start by helping our clients select the best drone or camera for the job. Also, we work hand-in-hand with them to find the most optimal flight path to get the most complete coverage of their asset. Then upon receiving the data on our cloud-based platform, we use our proprietary technology to create a highly detailed 3D model of the asset and by utilizing our AI, we detect and quantify the anomalies. The final deliverable package has a variety of data formats to make the life of the engineers and asset owners easier.
What are your thoughts on the future of AI in the asset-inspection sector? Will we see more generative AI in the way we analyze images, write programming codes, and run simulations?
Absolutely. We are just starting, and this is just the tip of the iceberg. Our goal is to keep improving our tech and moving into service life estimates and recommendations on how to fix damages or design new assets with the help of AI.
Were you always personally interested in technology and in becoming an engineer when you were a kid?
I have always loved new technologies. They were the shiniest toys for me growing up. The very first thing that I remember creating as a kid was a spaghetti bridge. Bridges have a special place in my heart, and I always wanted to be an engineer and build tools and technologies to change people’s lives. The impact of the job has always been a big motivator.
And lastly, is there any message related to the use of AI and drones in asset inspections that you would like to share with our readers?
Psychologists have known for decades that we tend to over-trust human skills and under-trust technology. This dynamic has played out for a very long time in asset inspection and management, resulting in the impediment of advancing new capabilities. We need to change our mentalities to be more open to new technologies in our industry as a whole.
Also, it is important to realize that human inspectors aren’t going away any time soon. As we’ve seen time and again, humans have incredible capabilities when it comes to the holistic assessment of a structure. But their job is changing rapidly.
Now, the modern inspector or engineer works with highly advanced technologies, such as autonomous drones, in a difficult field environment and is tasked with handling a wider range of data for decision support and asset management. Therefore, it is completely understandable why we need the help of AI solutions to advance infrastructure asset management and, as a result, optimize taxpayers’ money and improve public safety.